Transformers can be used for in-context linear regression in the presence of endogeneity
Best AI papers explained - A podcast by Enoch H. Kang - Thursdays
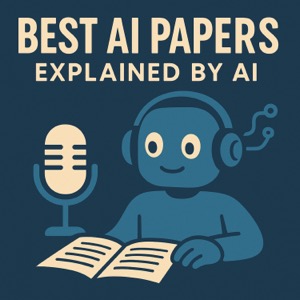
Categories:
This paper explores how transformers can be used for in-context linear regression in the presence of endogeneity. The authors demonstrate theoretically that transformers can effectively handle endogeneity by implementing instrumental variables (IV) techniques, specifically the two-stage least squares (2SLS) method, through a gradient-based approach that converges exponentially. They propose an in-context pretraining method with theoretical guarantees and show through experiments that trained transformers are robust and reliable, outperforming 2SLS in challenging scenarios like weak or non-linear IVs. The work extends the understanding of transformer in-context learning beyond standard linear regression and provides insights into extracting causal effects from these models.