Posterior Mean Matching Generative Modeling
Best AI papers explained - A podcast by Enoch H. Kang - Thursdays
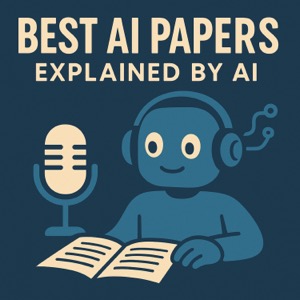
Categories:
This paper introduces Posterior Mean Matching (PMM), a novel generative modeling technique rooted in Bayesian inference. Unlike traditional diffusion models, PMM employs conjugate pairs of distributions to flexibly model diverse data types such as images and text. The core mechanism involves iteratively refining noisy data approximations through online Bayesian inference updates, with the convergence of the posterior mean to the true data sample forming the basis for generating new data. The authors demonstrate PMM's versatility by applying it to real-valued, count, and discrete data using Normal-Normal, Gamma-Poisson, and Dirichlet-Categorical models, respectively, establishing connections to stochastic differential equations (SDEs) and showing competitive performance in both image and text generation tasks.