Enough Coin Flips Can Make LLMs Act Bayesian
Best AI papers explained - A podcast by Enoch H. Kang - Thursdays
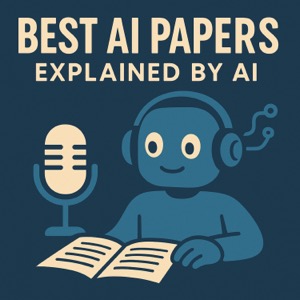
Categories:
This paper investigates whether Large Language Models (LLMs) utilize in-context learning (ICL) to perform reasoning consistent with a Bayesian framework. By using a simplified setting of biased coin flips and dice rolls, the authors analyze how LLMs update their internal probabilities based on provided examples. They find that LLMs often start with inherent biases (miscalibrated priors) but demonstrate behavior that broadly follows Bayesian updates when given sufficient evidence through ICL. The study indicates that deviations from true Bayesian inference primarily stem from initial poor priors rather than flawed updating mechanisms. Furthermore, the research suggests that attention magnitude has minimal impact on the Bayesian inference process in these models and that instruction-tuned models may exhibit shorter temporal horizons in their updates.