Bayesian Scaling Laws for In-Context Learning
Best AI papers explained - A podcast by Enoch H. Kang - Thursdays
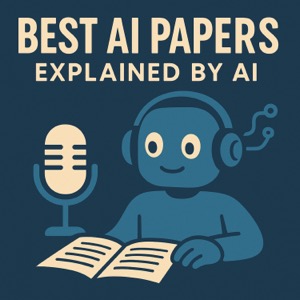
Categories:
This academic paper investigates whether in-context learning (ICL) in large language models (LLMs) functions like a Bayesian learner, aiming to explain why performance increases with more examples. The authors propose and derive novel Bayesian scaling laws that model the relationship between the number of in-context examples and prediction accuracy. Through experiments on synthetic data with toy models and real-world LLMs on various tasks, they demonstrate that their Bayesian laws accurately predict ICL behavior and offer interpretable parameters related to task priors and learning efficiency. The study suggests that post-training, like fine-tuning, primarily adjusts task priors rather than fundamentally altering the model's knowledge, which can explain why some suppressed behaviors might re-emerge through ICL.