Bayesian Concept Bottlenecks with LLM Priors
Best AI papers explained - A podcast by Enoch H. Kang - Thursdays
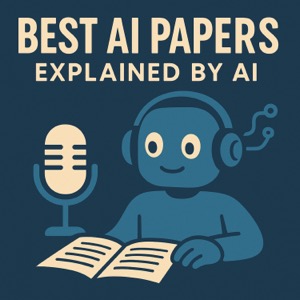
Categories:
This document introduces BC-LLM, a novel method for creating Concept Bottleneck Models (CBMs) that are both accurate and interpretable. Traditional CBMs rely on a predefined set of concepts, limiting their effectiveness and requiring significant human effort. BC-LLM addresses this by integrating Large Language Models (LLMs) within a Bayesian framework to iteratively discover relevant concepts. The LLMs serve as a concept extraction mechanism and provide prior information, enabling the model to explore a vast, potentially infinite, concept space. This approach is proven to provide rigorous statistical inference and uncertainty quantification, even when LLMs are imperfect, and experiments demonstrate its superior performance and robustness compared to existing methods across various data types.